Model-free neural fault detection and isolation for safe control
A new paper published in IEEE Control Systems Letters by researchers in the Reliable Autonomous Systems Lab at MIT (REALM) addresses the issue of detecting faults in robotic systems.
Authors: Kunal Garg; Charles Dawson; Kathleen Xu; Melkior Ornik; Chuchu Fan
Citation: “Model-Free Neural Fault Detection and Isolation for Safe Control,” in IEEE Control Systems Letters, vol. 7, pp. 3169-3174, 2023, doi: 10.1109/LCSYS.2023.3302768. https://ieeexplore.ieee.org/abstract/document/10210200
Abstract
In this work, we are addressing the issue of detection of faults in robotic systems. A sudden actuator fault in a safety-critical system can cause safety violations and lead to severe consequences. Existing fault-tolerant control (FTC) approaches normally focus on maintaining system performance and do not consider system safety. Control Barrier Functions (CBFs) have emerged as useful tools from control theory for providing safety guarantees for control systems. However, existing applications of CBFs either do not consider actuator faults or only consider the special case where it is known which actuator is faulty or the case when redundant actuators are present to maintain controllability even under faults and failures. In this letter, we address the problem of safe recovery under a more realistic scenario where it is completely unknown which actuator is faulty and when the fault occurs. We develop a novel model-free learning framework for an output-based neural fault-detector that detects when a fault occurs and in which actuator. Based on the learned functions, we propose a switching framework for automatically detecting and recovering from faults.
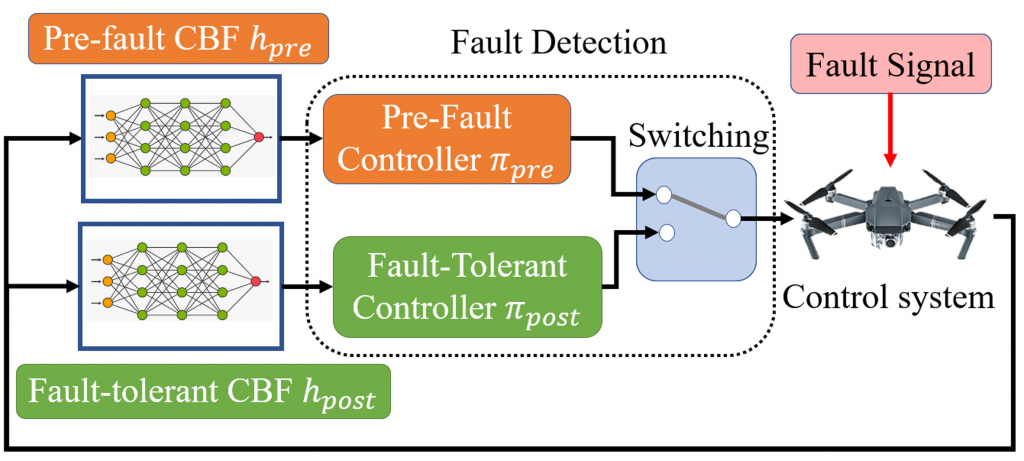