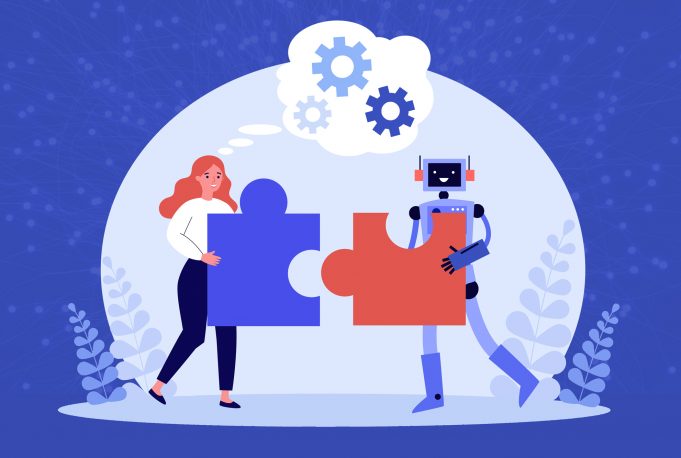
How to help humans understand robots
Scientists who study human-robot interaction often focus on understanding human intentions from a robot’s perspective, so the robot learns to cooperate with people more effectively. But human-robot interaction is a two-way street, and the human also needs to learn how the robot behaves.
Thanks to decades of cognitive science and educational psychology research, scientists have a pretty good handle on how humans learn new concepts. So, researchers at MIT and Harvard University collaborated to apply well-established theories of human concept learning to challenges in human-robot interaction.
They examined past studies that focused on humans trying to teach robots new behaviors. The researchers identified opportunities where these studies could have incorporated elements from two complementary cognitive science theories into their methodologies. They used examples from these works to show how the theories can help humans form conceptual models of robots more quickly, accurately, and flexibly, which could improve their understanding of a robot’s behavior.
Humans who build more accurate mental models of a robot are often better collaborators, which is especially important when humans and robots work together in high-stakes situations like manufacturing and health care, says Serena Booth, a graduate student in the Interactive Robotics Group of the Computer Science and Artificial Intelligence Laboratory (CSAIL), and lead author of the paper.
“Whether or not we try to help people build conceptual models of robots, they will build them anyway. And those conceptual models could be wrong. This can put people in serious danger. It is important that we use everything we can to give that person the best mental model they can build,” says Booth.
Booth and her advisor, Julie Shah, an MIT professor of aeronautics and astronautics and the director of the Interactive Robotics Group, co-authored this paper in collaboration with researchers from Harvard. Elena Glassman ’08, MNG ’11, PhD ’16, an assistant professor of computer science at Harvard’s John A. Paulson School of Engineering and Applied Sciences, with expertise in theories of learning and human-computer interaction, was the primary advisor on the project. Harvard co-authors also include graduate student Sanjana Sharma and research assistant Sarah Chung. The research will be presented at the IEEE Conference on Human-Robot Interaction.
A theoretical approach
The researchers analyzed 35 research papers on human-robot teaching using two key theories. The “analogical transfer theory” suggests that humans learn by analogy. When a human interacts with a new domain or concept, they implicitly look for something familiar they can use to understand the new entity.
The “variation theory of learning” argues that strategic variation can reveal concepts that might be difficult for a person to discern otherwise. It suggests that humans go through a four-step process when they interact with a new concept: repetition, contrast, generalization, and variation.
While many research papers incorporated partial elements of one theory, this was most likely due to happenstance, Booth says. Had the researchers consulted these theories at the outset of their work, they may have been able to design more effective experiments.
For instance, when teaching humans to interact with a robot, researchers often show people many examples of the robot performing the same task. But for people to build an accurate mental model of that robot, variation theory suggests that they need to see an array of examples of the robot performing the task in different environments, and they also need to see it make mistakes.
“It is very rare in the human-robot interaction literature because it is counterintuitive, but people also need to see negative examples to understand what the robot is not,” Booth says.
These cognitive science theories could also improve physical robot design. If a robotic arm resembles a human arm but moves in ways that are different from human motion, people will struggle to build accurate mental models of the robot, Booth explains. As suggested by analogical transfer theory, because people map what they know — a human arm — to the robotic arm, if the movement doesn’t match, people can be confused and have difficulty learning to interact with the robot.
Enhancing explanations
Booth and her collaborators also studied how theories of human-concept learning could improve the explanations that seek to help people build trust in unfamiliar, new robots.
“In explainability, we have a really big problem of confirmation bias. There are not usually standards around what an explanation is and how a person should use it. As researchers, we often design an explanation method, it looks good to us, and we ship it,” she says.
Instead, they suggest that researchers use theories from human concept learning to think about how people will use explanations, which are often generated by robots to clearly communicate the policies they use to make decisions. By providing a curriculum that helps the user understand what an explanation method means and when to use it, but also where it does not apply, they will develop a stronger understanding of a robot’s behavior, Booth says.
Based on their analysis, they make a number recommendations about how research on human-robot teaching can be improved. For one, they suggest that researchers incorporate analogical transfer theory by guiding people to make appropriate comparisons when they learn to work with a new robot. Providing guidance can ensure that people use fitting analogies so they aren’t surprised or confused by the robot’s actions, Booth says.
They also suggest that including positive and negative examples of robot behavior, and exposing users to how strategic variations of parameters in a robot’s “policy” affect its behavior, eventually across strategically varied environments, can help humans learn better and faster. The robot’s policy is a mathematical function that assigns probabilities to each action the robot can take.
“We’ve been running user studies for years, but we’ve been shooting from the hip in terms of our own intuition as far as what would or would not be helpful to show the human. The next step would be to be more rigorous about grounding this work in theories of human cognition,” Glassman says.
Now that this initial literature review using cognitive science theories is complete, Booth plans to test their recommendations by rebuilding some of the experiments she studied and seeing if the theories actually improve human learning.
This work is supported, in part, by the National Science Foundation.