ACC 2025 Workshop
Optimizing Across Scales: Multi-Fidelity, Multi-Modality, and Multi-Objective Approaches for Complex Systems
Date: July 7th, Monday, 2025
Time: 8:30 – 17:00, Mountain Standard Time
Location: Denver, Colorado, USA
Abstract
Given the growing ubiquity of things like autonomous vehicles, robots, and arrays of edge computing devices and sensors (collectively, known as the Internet of Things), the path forward in AI involves a progression from systems capable of addressing tasks within narrow, specialized domains—referred to as artificial narrow intelligence (ANI)—to systems with the ability to solve problems across multiple domains at or beyond human capability, known as artificial general intelligence (AGI) and artificial superintelligence (ASI). However, these superordinate scales of intelligence will not “emerge” naturally; instead, sophisticated optimization strategies are mandated to capacitate optimizing across multiple scales of intelligence, both within individual agents and across networks of interacting systems.
Optimization across scales is a fundamental aspect of intelligence, balancing trade-offs across various dimensions—from learning to synthesis to decision-making. For learning, the trade-off between accuracy and efficiency can be managed by leveraging models with multiple fidelity levels, ensuring effective usage of computational resources. In synthesis, achieving a balance between consistency and variability is critical when extracting and integrating information from multiple modalities. For decision-making, compromises among multiple objectives are often unavoidable due to conflicting goals, demanding ethical considerations. These multi-scale optimization strategies are prerequisites to unlocking the full potential of intelligent systems, enabling them to generate emergent forms of intelligence at higher, more complex levels.
Therefore, this workshop aims to explore multi-fidelity, multi-modality, and multi-objective optimization approaches, with a focus on how these methods can enhance efficiency, accuracy, consistency, variability, and ethicality. More specifically, tracking complex problems that involve:
* Multi-fidelity learning: Using both low-cost, approximate models and high-fidelity, resource-intensive models to efficiently explore solutions.
* Multi-modality integration: Combining diverse data sources, such as sensor data, imagery, and text, to inform decision-making processes.
* Multi-objective decision-making: Balancing conflicting objectives, such as speed versus accuracy or performance versus energy consumption.
By integrating insights from diverse fields—such as autonomous systems, smart cities, production lines and bioinformatics—we will discuss innovative ways to optimize across different scales, bridging the gap between theory and real-world applications.
Objectives
The workshop will provide a platform for researchers and practitioners to share methods, tools, and case studies that demonstrate the synergy between fidelity, modalities, and objectives. Attendees will learn how to apply these approaches to complex systems, where balancing trade-offs is critical to achieving robust, scalable solutions.
This workshop aims to:
1. Showcase recent advances in multi-fidelity, multi-modality, and multi-objective approaches across disciplines.
2. Demonstrate how these optimization strategies can improve the scalability, efficiency, and effectiveness of real-world systems.
3. Encourage collaboration between researchers and practitioners from different fields, including robotics, industry, bioengineering, and computer science.
Topics Covered
The workshop will feature topics including (but not limited to):
* Multi-fidelity modelling techniques for robotic systems and control applications
* Multi-modality data fusion for autonomous systems and human-robot interaction
* Multi-objective trade-offs in energy-efficient control, path planning, and decision-making
* Case studies on optimization in production lines, bioinformatics, smart cities, and transportation systems
* Tools and frameworks for multi-fidelity, multi-modality and multi-objective optimization
Schedule
Time | Speaker | Title |
8:30 – 8:50 | Workshop Organizers | Welcome and opening remarks |
8:50 – 9:30 | John Doyle | The Architecture of Brains: From Biology to Language, Technology, Sports, and Society |
9:30 – 10:00 | Coffee break | |
10:00 – 10:40 | Jonathan How | TBD |
10:40 – 11:20 | Dimitra Panagou | How to Enable Concurrent Safety and Resilience in Multi-Robot Networks? |
11:20 – 12:00 | Nisar Ahmed | Multi-modal Data Fusion with Humans on the Loop |
12:00 – 13:00 | Lunch break | |
13:00 – 13:40 | Andrew Clark | Control-Theoretic Approaches to Safety and Resilience of Learning-Enabled Autonomous Systems |
13:40 – 14:20 | Ran Dai | Tensor-based Koopman Operator for Optimal Control |
14:20 – 15:00 | Mengxue Hou | Assured Abstraction for Hierarchical Robotic Planning |
15:00 – 15:30 | Coffee break | |
15:30 – 16:10 | Yongxin Chen | TBD |
16:10 – 16:50 | Panel discussion | TBD |
16:50 – 17:00 | Workshop Organizers | Conclusion and closing remarks |
Invited Speakers
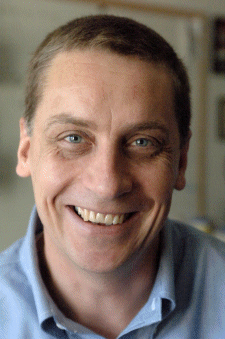
Jonathan How
Massachusetts Institute of Technology
Title: TBD
Abstract: TBD
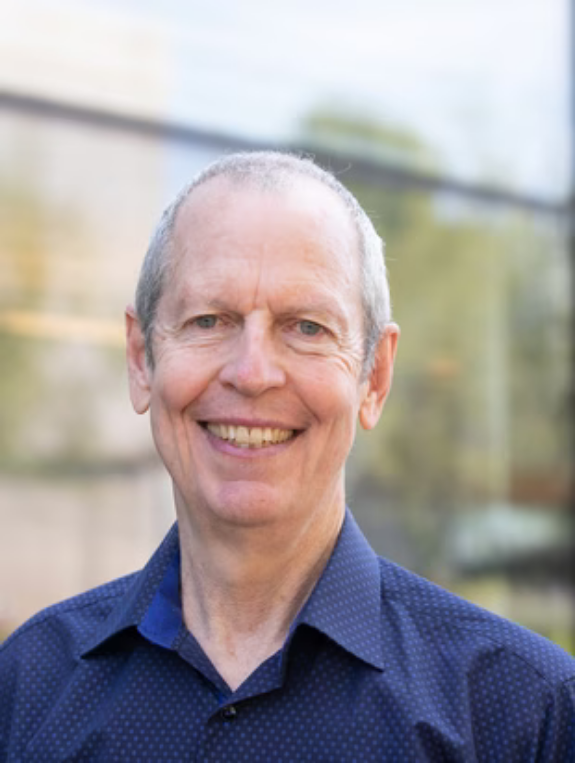
John Doyle
California Institute of Technology
Title: The Architecture of Brains: From Biology to Language, Technology, Sports, and Society
Abstract: The human brain’s evolution, enabling language, technology, medicine, and society, encompasses bewilderingly diverse details and systems. Yet, all these advancements are facilitated by a shared Universal Layered Architecture (ULA). ULAs are pervasive because virtualization allows builders and users—including scientists and engineers—to grasp only fragments, while the architecture itself persists, evolves, and propagates. While ULAs usher in remarkable triumphs, they also harbor catastrophic vulnerabilities, exposing systems to pathogens, cancer, patriarchy, and the resulting pandemics and wars. ULAs thus remain perilously misunderstood. Beginning with sensorimotor control, this talk will outline ULA concepts such as Diversity-Enabled Sweet Spots (DeSS) and “constraints that deconstrain” via layers, levels, stages, the bowtie and hourglass models, and new laws on efficiency and robustness. Theory demonstrates that ULAs are necessary in overcoming biology’s hardware layer constraints on sensing, communications, computing, and actions—such as sparsity, locality, saturation, and delays. This nascent theory also suggests enhancements in both understanding and design of ULAs. Examples range from cellular to societal scales, including successful animal models of social architectures from which humans could derive valuable lessons.
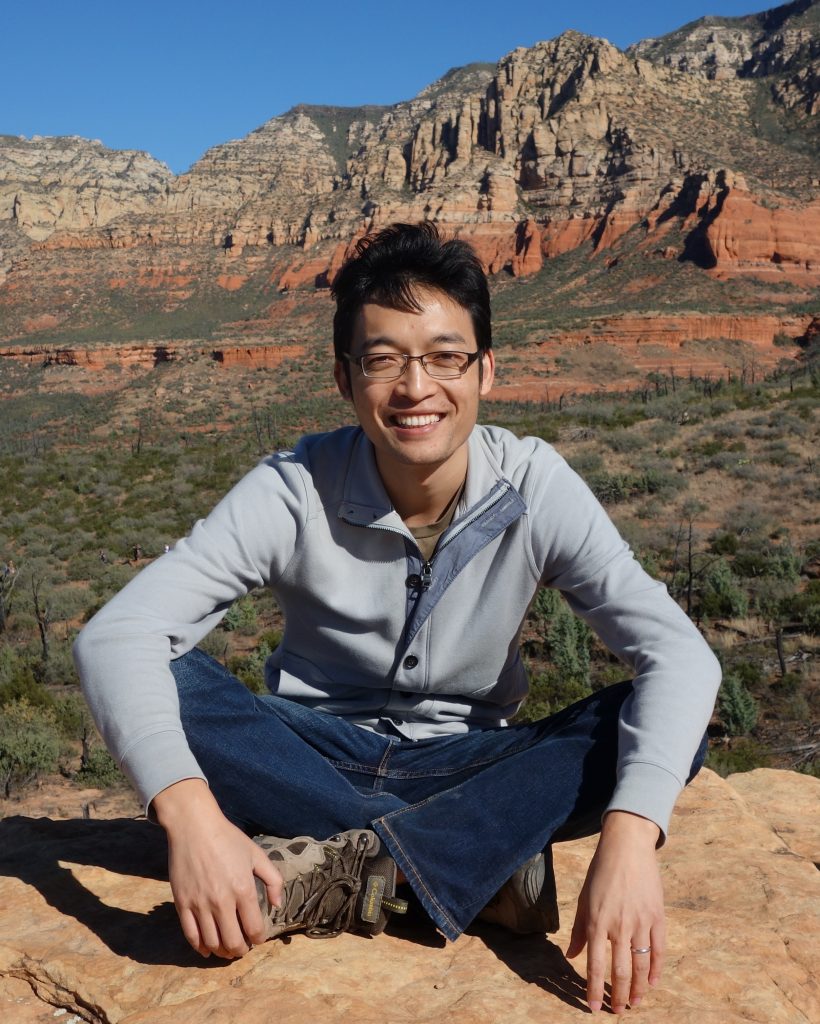
Yongxin Chen
Georgia Institute of Technology
Title: TBD
Abstract: TBD
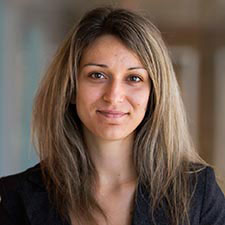
Dimitra Panagou
University of Michigan
Title: How to Enable Concurrent Safety and Resilience in Multi-Robot Networks?
Abstract: The proliferation of cyberattacks in today’s world has sparked significant interest during recent years in the resilience of networked systems against failures and attacks. A plethora of distributed estimation and control approaches have been developed, that often focus on either attacks that target the “cyber” domain (e.g., the information shared via communication or acquired via sensing), or on attacks that target the “physical” domain (e.g., the actuators or the entire plant/network). Despite tremendous progress, there are still open problems, including but not limited to how we can obtain less conservative models of the attacks (beyond worst-case assumptions), and how we can ensure the safe operation of the agents despite the effects of the attacks. This talk presents an overview of our recent work on resilient multi-robot networks against “cyber” adversaries (Byzantine agents) and “physical” adversaries (risk-averse and risk-taking agents), and some highlights of our ongoing work on learning and counteracting adversarial behavior in multi-agent/multi-robot systems.
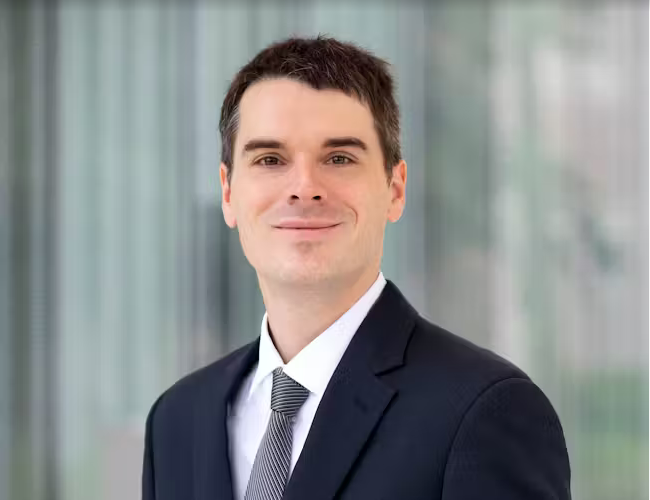
Andrew Clark
Washington University in St. Louis
Title: Control-Theoretic Approaches to Safety and Resilience of Learning-Enabled Autonomous Systems
Abstract: Autonomous systems in applications including robotics, manufacturing, and driverless vehicles are required to perform complex missions while also ensuring safety of the systems, supporting infrastructures, and human bystanders. While learning-based methods have shown substantial progress in achieving these objectives, they face challenges including scalability to high-dimensional systems, the need for verifiable safety, and robustness and resilience to naturally-occurring faults, deliberate adversarial attacks, and deviations between training and operational environments. This talk will present recent research advances towards addressing these challenges. First, we will present a control-theoretic framework for safety verification of learning-enabled autonomous systems. This framework utilizes techniques from algebraic geometry and set-theoretic methods in control to provide scalable and exact safety and reachability criteria for neural network controllers. Second, we will extend our framework to provide safety and performance of systems that experience sensor and actuation failures.
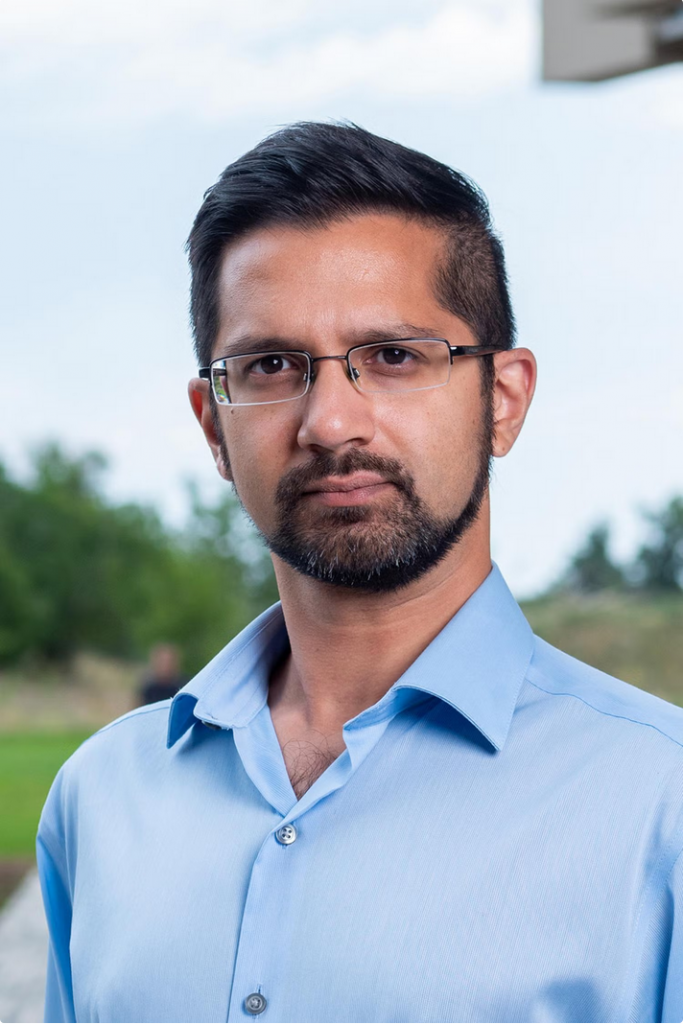
Nisar Ahmed
University of Colorado Boulder
Title: Multi-modal Data Fusion with Humans on the Loop
Abstract: From the development of foundational state space estimation tools like the Kalman filter to state of the art machine learning methods for sensor fusion and decision making, probabilistic models and reasoning algorithms are the “lingua franca” for modern robotics and autonomous systems. Probabilistic AI and learning have evolved in new and exciting ways to tackle fundamental research questions for current and future systems that require multimodal data fusion, including with human information sources. I will highlight recent work on human-machine/robot interaction for collaborative information gathering and reasoning, using probabilistic state estimation and decision-making algorithms. These methods plug seamlessly into existing multimodal data fusion architectures and can exploit the capabilities of human collaborators to provide information-rich semantic data via user-friendly interfaces that are typically “out of band” for autonomous platforms. Moreover, these methods come with built-in probabilistic mechanisms that allow them to stay robust against erroneous or unexpected human inputs. This leads to generalizations of probabilistic data association techniques that can be extended to human input modalities like semantic data or hand-drawn sketches. Applications to aerospace applications such as integrated surveillance/reconnaissance, wilderness search and rescue, and remote space exploration will be demonstrated and discussed.
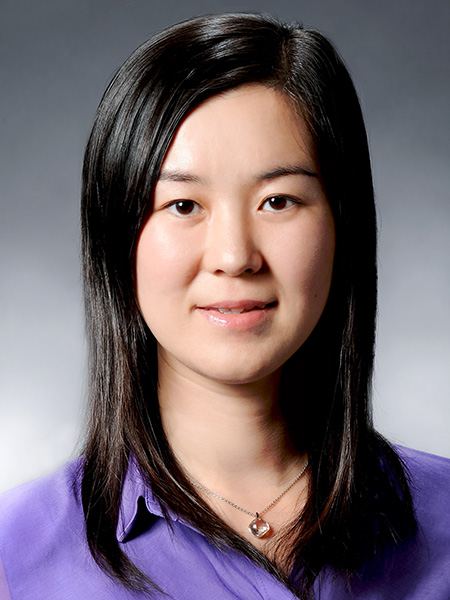
Ran Dai
Purdue University
Title: Tensor-based Koopman Operator for Optimal Control
Abstract: Koopman operator theory serves as a comprehensive linearization mechanism for analyzing and controlling complex nonlinear systems by projecting them into a higher-dimensional functional space. In practice, this involves the finite-dimensional approximation of the inherently infinite-dimensional Koopman operator using a selected set of observable functions. The efficacy of this method relies on the precision of these approximations, which typically enhances with the inclusion of more observables. However, such expansions greatly increase the storage demands and computational load, especially for systems with high dimensionality, thus impeding practical implementations. Our research addresses these challenges by employing tensor-based approaches to reconfigure the Koopman operator, effectively mitigating the curse of dimensionality. This leads to a robust linear prediction model that utilizes our tensor-structured Koopman operator. Additionally, we introduce an innovative optimal control strategy derived from this tensor-based Koopman linearization model. This strategy converts the nonlinear dynamics and constraints into a linear framework, which allows for employing state-of-the-art convex optimization to facilitate real-time computation.
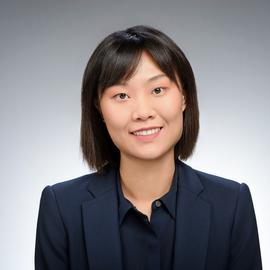
Mengxue Hou
University of Notre Dame
Title: Assured Abstraction for Hierarchical Robotic Planning
Abstract: To enable a smart and autonomous system to be cognizant, taskable, and adaptive in exploring an unknown and unstructured environment, robotic decision-making relies on learning a parameterized knowledge representation. However, one fundamental challenge in deriving the parameterized representation is the undesirable trade-off between computation efficiency and model fidelity. This talk addresses this challenge in the context of underwater vehicle navigation in unknown marine environments. To improve fidelity of the reduced-order model, we develop a learning method to generate a non-Markovian reduced-order representation of the environmental dynamics. Such abstraction guarantees to improve the modeling accuracy. Further, taking advantage of the abstracted model, we develop a Large-Language-Model-guided hierarchical planner to translate human specified missions directly to a set of executable actions with low computation cost.
Organizers
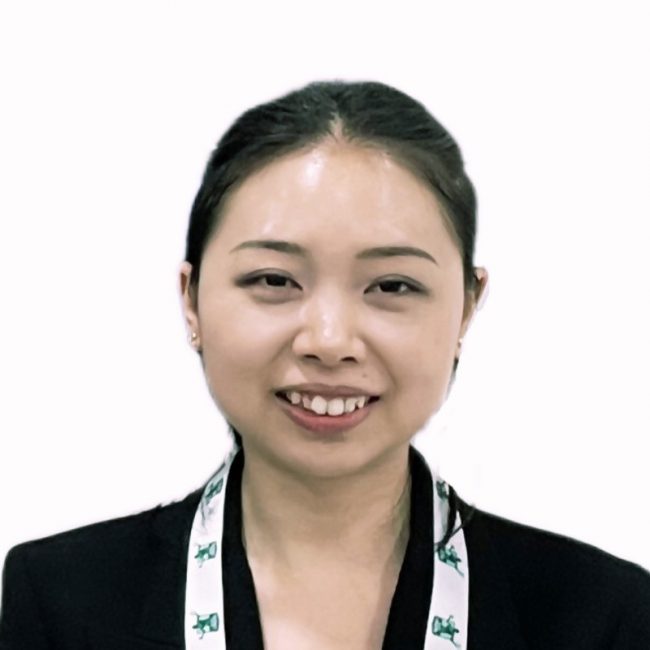
Yingke Li
Massachusetts Institute of Technology
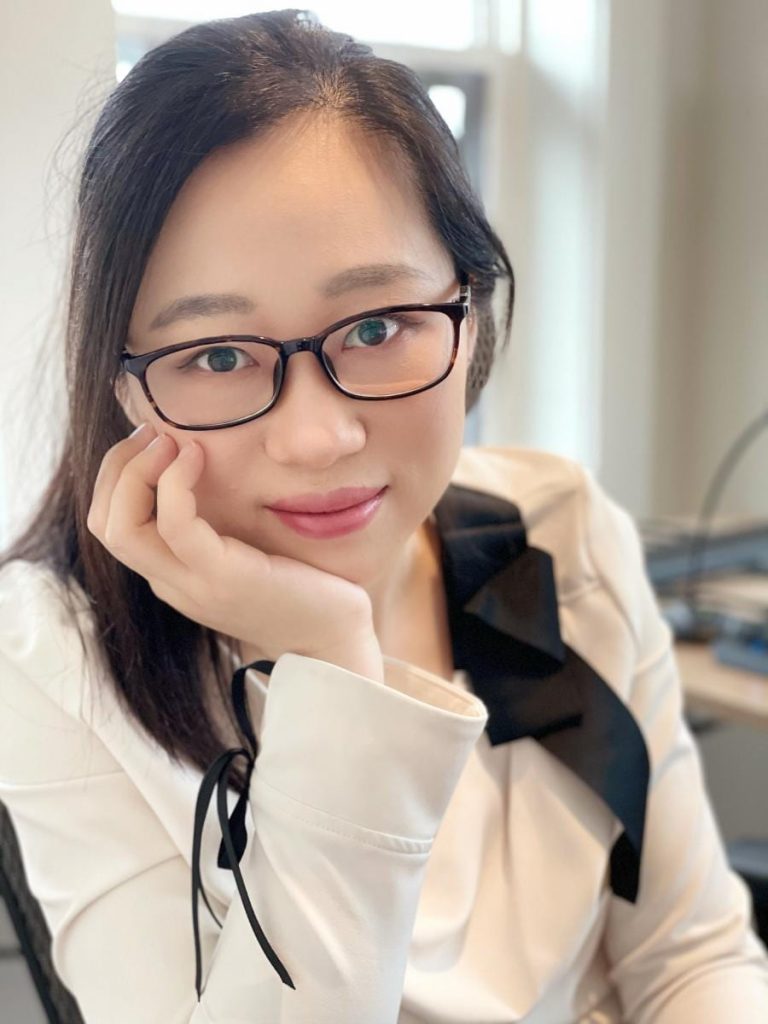
Chuchu Fan
Massachusetts Institute of Technology
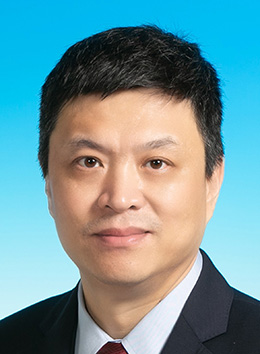
Fumin Zhang
Hong Kong University of Science and Technology