Reinforcement Learning for Space Situational Awareness Sensor Tasking
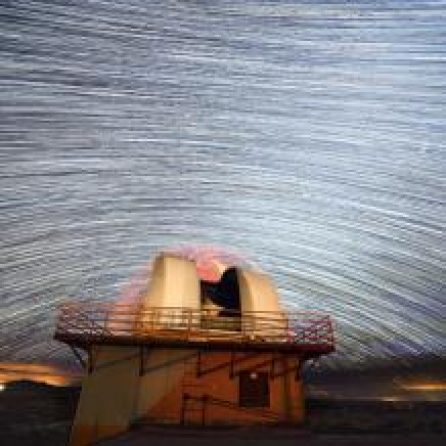
With the recent growth of new satellites launched, it is more imperative than ever before to keep track of these orbiting objects for collision avoidance and efficient use of space. The traditional space situational awareness (SSA) sensors are limited in field of view and slew rate, which means that the sensors can only select a few objects to track overhead at a time. Efficient and optimal tasking of these sensors for better SSA is needed to maximize the information gained from tracking the objects. In this project we look at using reinforcement learning algorithms and compare them to myopic methods for a discretized observation and action space model in several orbit regimes. We are also expanding the work to multiple sensors and space-based sensors.
Image: Dan Jang / MIT