Geosynchronous Satellite Pattern-of-Life Characterization
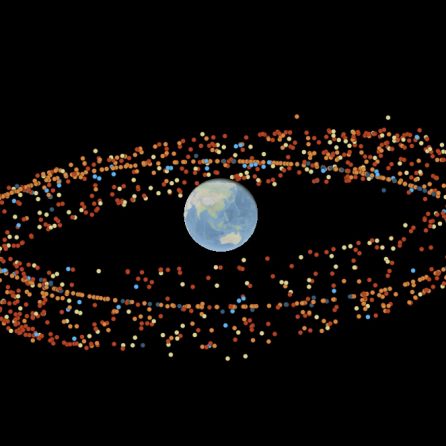
Unlike satellites orbiting the Earth at lower altitudes, those in the geosynchronous orbital regime (GEO)—a ring around the Earth more than 30,000 kilometers above its surface—are designed to be relatively long-time residents of the space domain, with decades-long operational lifetimes. During their time in GEO, satellites are most often commanded to perform maneuvers that keep them near a particular position in the Earth-centered, Earth-fixed (ECEF) reference frame. Less often, satellites shift their position in ECEF space, abandon their station, or remove themselves from GEO entirely as part of a retirement maneuver. In traditional space situational awareness analysis, such behaviors are deduced from high-dimensional historical orbital element data. As the GEO environment becomes more populated and more observational data become available with the advent of higher-fidelity observational tools in the commercial sector, GEO satellite behavior analysis will continue to mature into a big data problem. A research thrust within ARCLab is to use machine learning techniques to characterize GEO satellite patterns of life (PoLs): models of historical satellite behavior using sequences of behavioral modes separated by nodes during which satellites shift from one behavioral mode to another. Satellite PoL characterization offers the opportunity to succinctly describe satellite behavior by defining behavioral modes with relevant parameters, such as maneuver periodicity, magnitude, and direction for station-keeping modes or drift rate for longitudinal-shift modes. Satellite PoL characterization can contribute to real-time anomaly detection, orbital slotting adherence analysis, and PoL mode-shift prediction.
Software tools associated with this work can be accessed in the MIT ARCLab GEO Toolbox on GitHub.
Image: SatelliteDashboard.org