AI-Assisted Optimization of Schedules
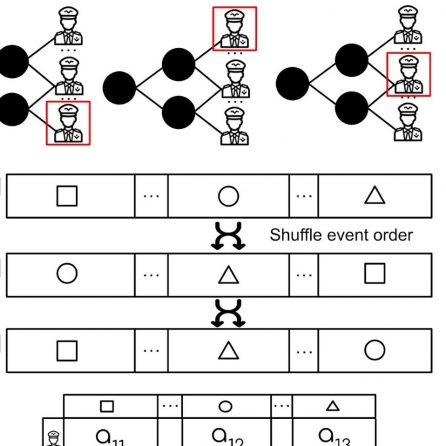
Air Force flight and training scheduling is a labor-intensive and largely manual process. Complex training requirements and dependencies, operational constraints, numerous qualifications, and unforeseen missions confound the schedule development process. In this project, we develop deterministic and robust optimization formulations for the Air Force training scheduling problem. We account for the propagation of disruptions, incorporate user preferences, and identify metrics and objective functions to compare candidate schedules.
More information
Chris Chin’s talk at INFORMS 2020
MIT News article on the project
NICE: Robust Scheduling through RL-Guided Integer Programming
People
Christopher Chin, Daniel Liu, Jason Luo, Siddharth Nayak, Hamsa Balakrishnan
Collaborators/alums: Carson Smith, Luke Kenworthy, Matt Koch, Karthik Gopalakrishnan, Kendrick Cancio, Ronisha Carter, Allison Chang, Jessamyn Liu, Travis Smith, Mike Snyder
Publications
- S. Nayak. “Learning-based Scheduling”. SM Thesis, MIT, 2022. [link]
- C. Smith. “Attention-based Learning for Combinatorial Optimization”. MEng Thesis, MIT, 2022. [link]
- L. Kenworthy, S. Nayak, C. Chin, and H. Balakrishnan. “NICE: Robust Scheduling through RL-Guided Integer Programming,” Proceedings of the Thirty-Sixth AAAI Conference on Artificial Intelligence (AAAI-22), February 2022. [link]
- M. Koch. “Air Force Crew Scheduling: An Integer Programming Approach”. SM Thesis, MIT, 2021. [link]
- C. Chin. “Disruptions and Robustness in Air Force Crew Scheduling”. SM Thesis, MIT, 2021. [link]
Research was sponsored by the United States Air Force Research Laboratory and was accomplished under Cooperative Agreement Number FA8750-19-2-1000. The views and conclusions contained in this document are those of the authors and should not be interpreted as representing the official policies, either expressed or implied, of the United States Air Force or the U.S. Government.